Humans are imperfect. We can strive for perfection but doing so takes conscious effort. In the context of the workplace, imperfections can lead to bias and discrimination which the removal of, no matter how it manifests itself, is neither simple nor easy and also requires conscious effort.
Some suggest that one solution is to use computers and automation to make decisions since they are unemotional and binary in their inputs and outputs – after all, they’re blind to anything other than data. Artificial intelligence (AI) is the most recent development in this line of thinking as it offers, so the theory goes, an ability to learn and improve on a continuous basis.
But while AI can undoubtedly be applied and is relevant in certain fields, it is not necessarily best placed to make decisions regarding people, diversity, equity, and inclusion. In fact, under specific circumstances, it can cause more harm than good.
Potential for bias
The problem was summarized recently by Agbolade Omowole, CEO of Mascot IT Nigeria, at the World Economic Forum in a report entitled Research shows AI is often biased. Here’s how to make algorithms work for all of us. He outlined, in very precise terms, how existing human bias is all too often transferred by developers to AI systems which, in turn, become fundamentally biased themselves.
Omowole isn’t suggesting the deliberate ‘designing in’ of bias during the build process but rather that unconscious and unintended bias can seep into programming. And he offered two good examples of unintended consequences of this: one, at Amazon, where a system to review job resumes led to women being discriminated against for technical roles; and another involving San Francisco lawmakers who voted against the use of facial recognition as they believed it is prone to errors when used on women or people with dark skin.
James Manyika, Jake Silberg, and Brittany Presten made a similar point in a paper published on the Harvard Business Review, What Do We Do About the Biases in AI? They said that human biases are well-documented and demonstrable. They also recognized that societies are starting to wrestle with just how much these biases can make their way into AI systems.
So, from a position nearly 35 years on from when the very first AI systems began to be deployed, algorithms have in recent years become considerably more complex and sophisticated. However, the same challenge exists – AI can help identify and reduce the impact of human biases, but it can also make the problem worse by ‘baking in’ and deploying biases at scale in sensitive application areas.
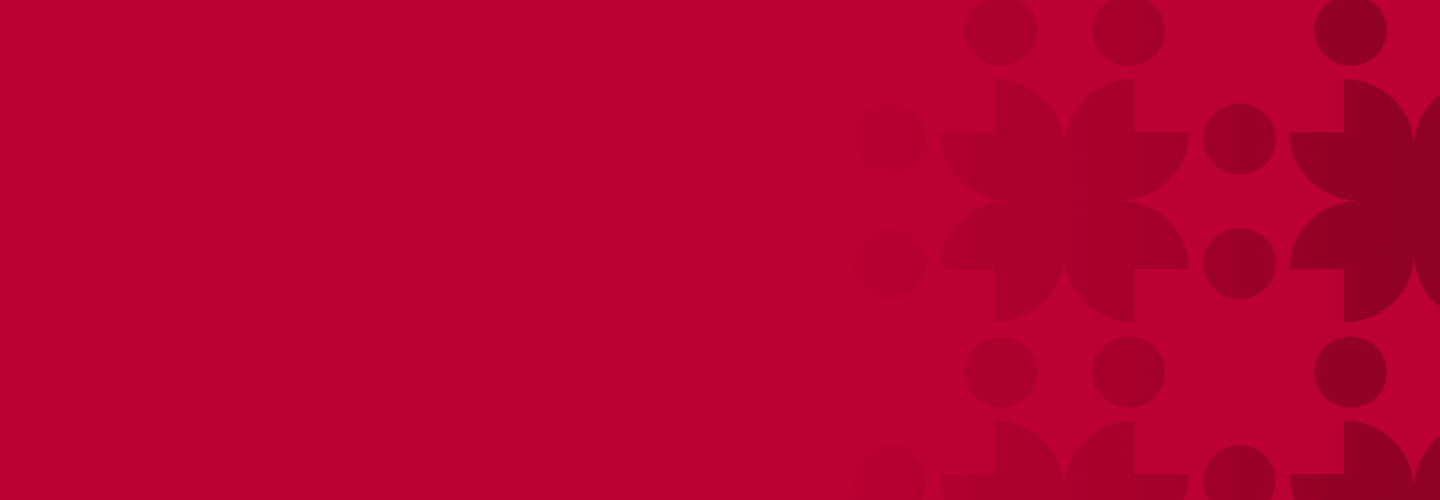
AI can help identify and reduce the impact of human biases, but it can also make the problem worse by ‘baking in’ and deploying biases at scale.
It’s a matter of principle for the three authors of the paper that at a time when many companies are looking to deploy AI systems across their operations, they need to be acutely aware of those risks and work to reduce them as a priority.
Risk of AI-enabled reproduction of existing bias
The problem of bias is very real and represents injustice against a person or a group. When it comes to AI, existing human bias can be transferred to systems because technology and software applications will only ever be as good – or as bad – as the developers behind it. This is especially so with the larger corporate developer as there may be no one in a position to offer an alternative perspective to unconscious biases that often inadvertently promote, for example, white males over others. In essence, AI systems won’t know any better and so will perpetuate any bias built into their programming.
But there is a solution. Organizations can hire diverse people to devise correct processes which are overseen by a chief diversity officer who checks software that is in development for bias, create applications and processes that remove bias, and that will bring benefits in the future.
But for now, we are still left with a major problem – machine learning and AI is invariably based on existing, and therefore biased, data.
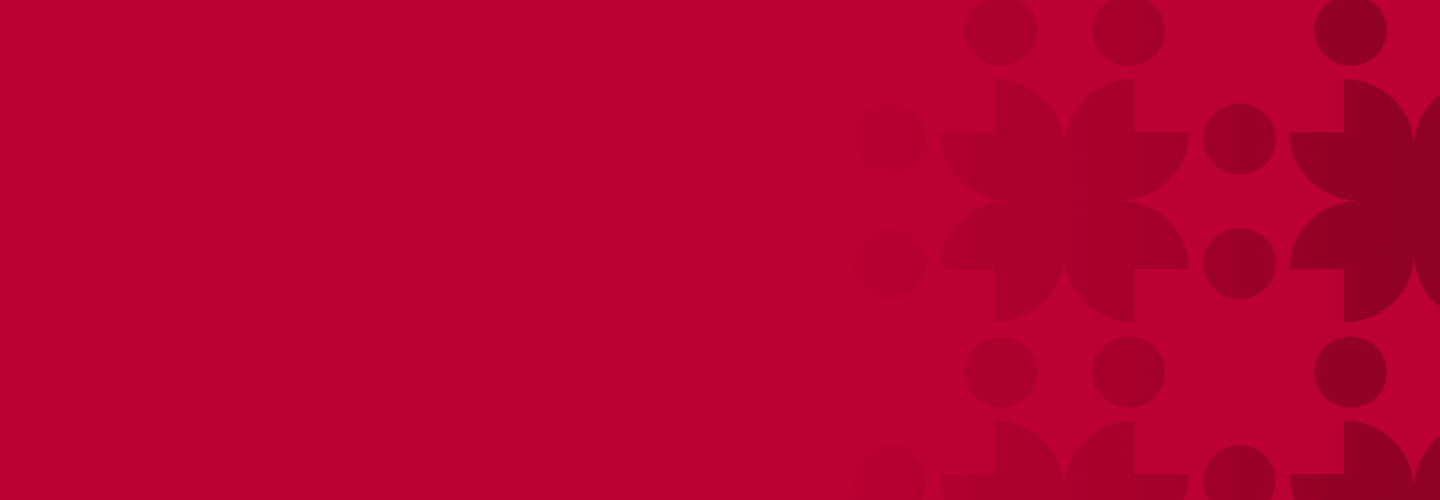
Programming an AI with data based on existing trends, observations and behaviors will undoubtedly, despite the efforts of the organization, still perpetuate bias – a case of ‘garbage in, garbage out.
So, to the extent that there is already bias embedded in current data – and there will be bias because organizations generally lack the diversity of voices and talent representation within data that is used – the only work around is to seek out data sets for AI systems that is grounded on diversity and inclusion.
To reiterate the point made earlier by Omowole, we can look at the AI-based conversational Twitter chatbot, Tay, that Microsoft released in 2016. It was supposed to interact with people through tweets and direct messages. But because it was learning from Twitter, it was replying with highly offensive and racist messages within a few hours of its release, because it could only learn from anonymous public data. This wouldn’t have happened if its core knowledge and learning was based on the principles of diversity and inclusion.
The popular conversational AI ChatGPT, which continually learns from those using the tech, creates more subtle examples of discrimination and stereotyping. The New York Times journalist Emma Grillo wrote about her experiences with the chatbot. When she asked it whether she should wear a white dress to a wedding, it suggested that she check with the bride if this would be acceptable. Grillo notes that this would have been difficult given that at this particular wedding there was no bride – only two grooms!
She also found that ChatGPT’s suggestions for work wear were clouded by bias. ‘A mid-thigh dress,’ it claimed, ‘may distract the interviewer’s attention.’ In a similar experiment of my own, ChatGPT proposed that a knee-length, V-neck dress might be appropriate attire for a job interview as long as it is not ‘too revealing’ and that a blazer or cardigan could be worn to cover my shoulders.
What AI systems ‘know’ is wrong
Interested in learning more about the biases inherent within AI, I asked ChatGPT to list the top three soft skills that a woman should practice if she wants to become a senior leader in the technology sector. The chatbot suggested collaboration and innovation, but also put forward technical acumen:
‘While soft skills are essential for success in any leadership role, women in the technology sector may also need to demonstrate a strong understanding of technical concepts and processes to be effective leaders.’
How about for a man? ChatGPT responded with adaptability, communication and collaboration.
Giving ChatGPT the benefit of the doubt, I asked it to regenerate the response three further times. The only new suggestion was strategic thinking. It appears that for a man working in tech, the AI assumes they will have mastered technical acumen without any prompting – for a woman though, it’s time to upskill (apparently).
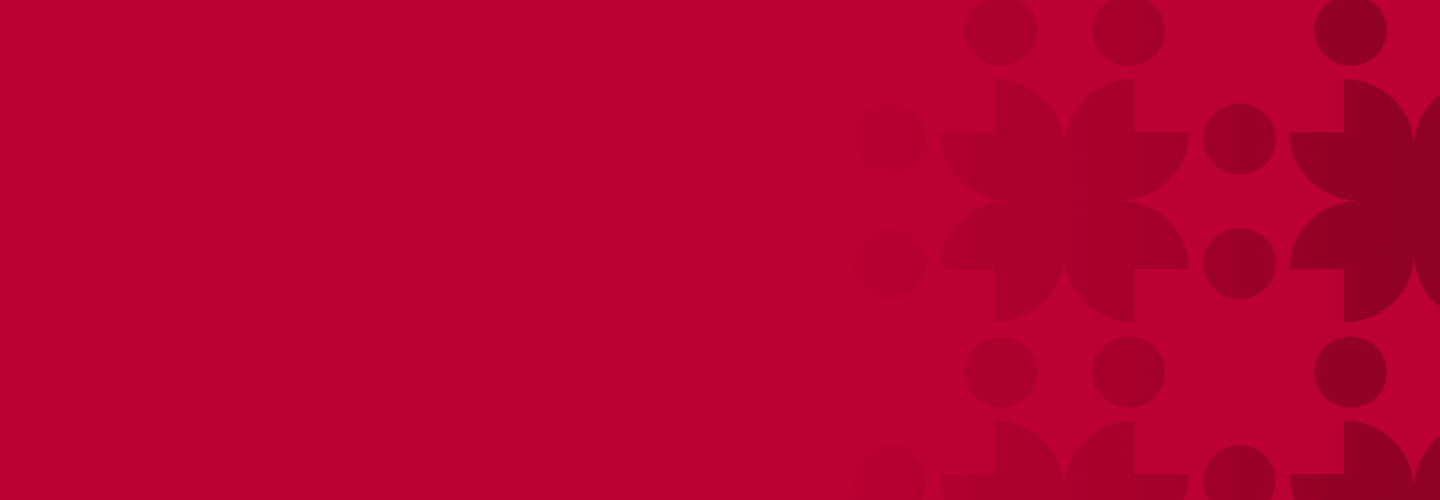
It appears that for a man working in tech, the AI assumes they will have mastered technical acumen without any prompting – for a woman though, it’s time to up-skill (apparently).
It could be argued that ChatGPT is simply reflecting back the biases that already exist – but with AI becoming more dominant in modern society, we should surely be creating technologies to challenge these biases instead of finding new ways to preserve them?
Similar scenarios can happen with HR systems where patterns of bias and discrimination are embedded into operational data. Systems may think – and determine – that women should only ever be employed as secretaries or work in HR functions, because that is what the bias data will have them believe. The same system may consider men as destined to become highly paid CEOs.
Fundamentally, algorithms in AI systems will only ever replicate what they ‘know’. A compromised system that considers comments from employee surveys, trends relating to promotion, race and recruitment will only ever reinforce the status quo.
Conscious meets unconscious bias
As the DE&I agenda continues to gain momentum, AI technologies are undoubtedly learning about the dangers of allowing biases to remain unchecked. Unfortunately, this isn’t enough – just as it isn’t enough for organizations to resolve the myriad issues around inequity and lack of diversity by simply ‘knowing’ that they exist. How can we expect biases to disappear without proactive strategies to tackle them? Consciousness doesn’t equal change.
For example, when asked what HR issues a woman should be aware of when joining a small engineering team, ChatGPT suggested that ‘women in male-dominated fields like engineering can sometimes face bias and stereotyping from their colleagues.’
Despite not being informed of the gender of the other team members, the bot ‘assumed’ that the rest of the team would be male. Based on historical data, you could argue that this is a logical assumption. But without challenging the inequities that have resulted in this data set, the chatbot is reinforcing them as ‘the norm’.
When I confronted ChatGPT about the assumption, it apologized. Rather than implying anything about the gender of the people being referred to, it said that it uses the pronoun ‘he’ for ‘simplicity and brevity’ – an unconvincing justification for the use of uninclusive language. It went on to state:
‘It is important to be mindful of the diversity and inclusivity of all team members, regardless of gender, race, or any other factors.’
It is clear that ChatGPT is conscious that biases exist and yet by using men as the default gender, it perpetuates them. And in doing so, biases remain – in many cases – unconscious.
Opt for reliability
Of course, none of this is about denying the potential for AI systems, but they can exhibit limitations if only biased data is fed into them.
However, there are pockets of reliable data, such as data held by EDGE on EDGE Lead Certified organizations that can safely and reliably be used to train AI-based diversity and inclusion solutions. This is because the organization will have been independently verified and the data it generates will be as close as it can be to being unbiased.
And while the quality of data that can be trusted is difficult to find outside of independently verified certification systems that uphold the highest standards in diversity, equity and inclusion, we are seeing that the pool of EDGE Lead Certified organizations is growing. This means that the pool of data that can be trusted is similarly growing and becoming more widely available.
In summary
AI systems do have a place within organizations, and they certainly have role in running equity processes. But organizations need to be alive to biases held by software developers and also, the potential for inherent bias of the data used in processes. This will make the difference between AI reinforcing the bias in a process, or effectively ‘de-biasing’ them.